Predicting drug-resistant Tuberculosis
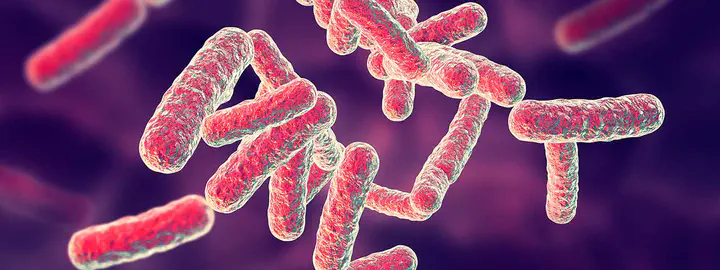
Systems Genomics Modelling of Drug Resistance in Mycobacterium tuberculosis
Multi-drug resistance presents a growing challenge to global tuberculosis (TB) control efforts, with a particularly pressing concern in developing nations where robust disease surveillance and monitoring are lacking. Nepal is one of the countries with a high TB burden. TB is caused by an airborne bacterium, Mycobacterium tuberculosis (Mtb). Treatment for TB involves antibiotics, however, some Mtb becomes resistant to these drugs, forming drug-resistant TB, which is rapidly increasing and poses a major health threat. The emergence of drug resistance TB is linked to specific mutations in Mtb genome. However, the genetic foundations of drug resistance in TB are not fully understood. The existing catalog of well-characterized Mtb drug-resistant mutations falls short in elucidating numerous instances of drug-resistant TB. Identifying new drug-resistant TB mutations is challenging given the genetic diversity of TB and the complex mechanisms driving the drug resistance. To address these challenges, here, we aim to develop a machine learning method to predict drug-resistant TB leveraging a large pool of TB genomic data. Additionally, we will explore the underlying metabolic adaptation in drug-resistant TB using genome-scale metabolic modeling.
Research Funded by